For many lecturers, that eureka moment when their students finally grasp a difficult topic is priceless. To make sure students get to that point, every teacher strives to make learning an enjoyable and enriching experience for those under their instruction. Recently, four DigiPen (Singapore) faculty members — assistant professors Dr. Christopher Teoh and Dr. Sayed Irfan, as well as lecturers Priyanka Bhoyar and Wang Zhiyuan — took this motivation for teaching a step further. Inspired to help their students learn better, they worked together to publish Numerical Machine Learning, a textbook that helps bridge the gap between mathematics theory and practice.
With several machine learning textbooks already in the market, what inspired Christopher, Irfan, Priyanka, and Zhiyuan to publish their own? “The idea for this project was birthed back in May 2022,” Zhiyuan says. He and Irfan were talking about their machine learning class and how difficult it was to find any existing numerical examples to explain the concepts touched on in their course materials. “The various textbooks in the market had a strong emphasis on using abstract mathematics to discuss the theories of machine learning algorithms, instead of simpler, numerical examples,” Zhiyuan explains. In order to make these topics more easily understood for their students, Zhiyuan and Irfan had resorted to creating their own examples. Midway through their discussion, a thought occurred to both of them: Since there was clearly a need in this area, why shouldn’t they be the ones to fulfil it? Zhiyuan and Irfan brought their idea to Christopher and Priyanka, and the four of them agreed to embark on the project together.
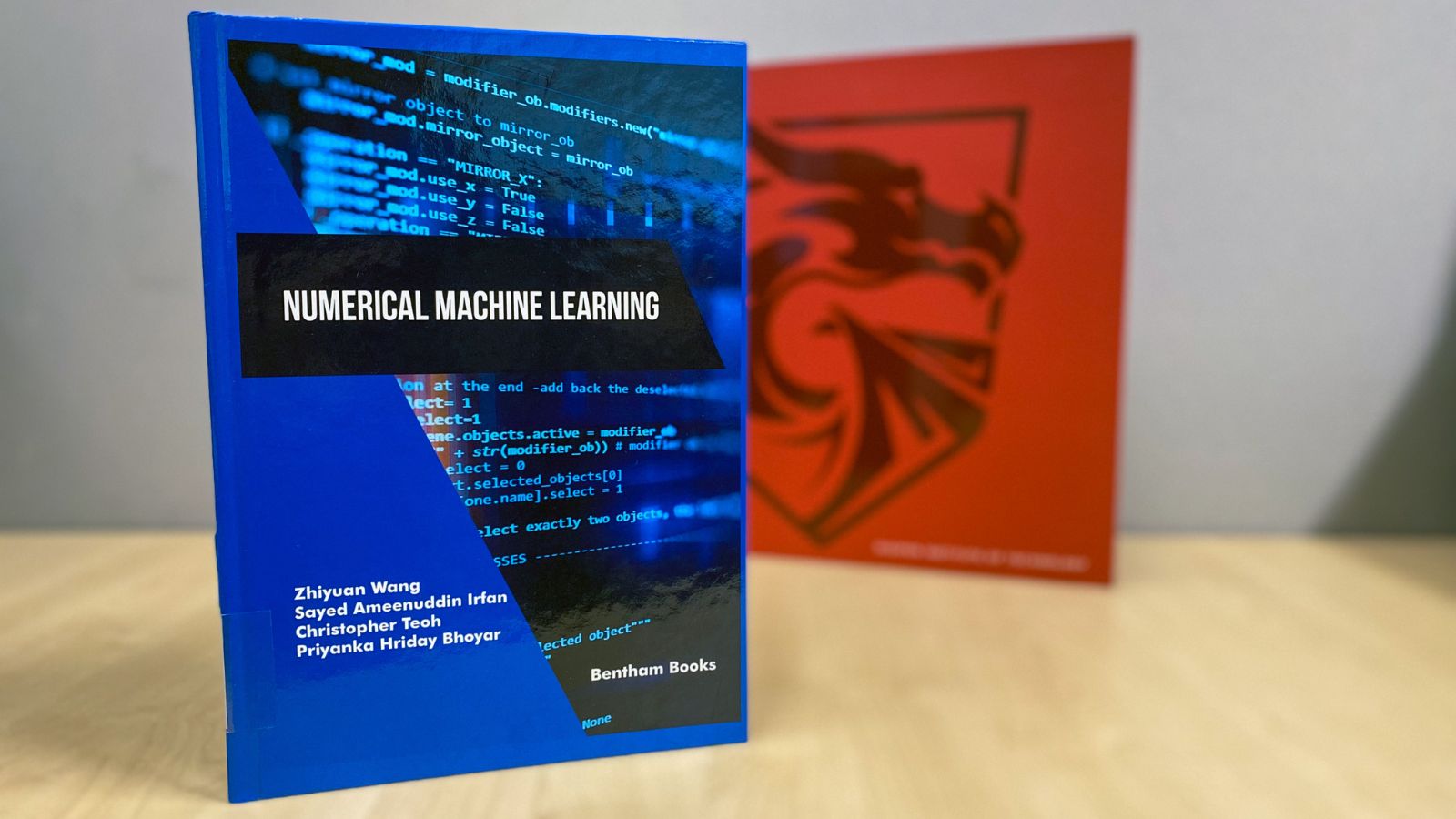
The four faculty members say there are many benefits to using numerical examples to understand complex machine learning theories. For starters, concrete numerical examples — as opposed to abstract ones — help readers see how the algorithms work in practice, making it easier to understand the concepts and ideas behind the theories. Additionally, working through numerical examples also encourages readers to approach different types of problems in a more systematic way, honing their critical thinking skills in the process. Seeing how machine learning algorithms behave using actual data can also help readers identify some common pitfalls to avoid in their own work. Working through numerical examples is thus an effective way to learn about machine learning, as it helps students develop the necessary skills and knowledge to apply it.
Once the team had an overview and idea of the topics they would include in the textbook, they started pitching to various scientific publishers and eventually heard back from Bentham Science. They then started fleshing out the content, splitting the workload by writing two chapters each. The final manuscript was completed at the end of 2022 and eventually published earlier this year. The team even started using their own textbook to teach their newest cohort of students this past May. “That was the most fulfilling moment for all of us,” Zhiyuan says. “We had come full circle.”
The experience of publishing a book made the team realize how well they worked with one another. Inspired to do more, the four colleagues — together with fellow DigiPen (Singapore) lecturer Anne Yu — decided to establish a research team, which they are calling the Artificial Intelligence Research and Computational Optimization (AIRCO) laboratory. As its name suggests, AIRCO is dedicated to pioneering advancements in artificial intelligence (AI) and computational optimization in various domains. The team has cited a wide variety of interests spanning applied AI in sustainability, generative AI, multi-objective optimization, metaheuristic algorithms, data engineering, and more. The AIRCO members hope to tap on each faculty’s varied background and expertise to advance research and push for more breakthroughs in the field.
Numerical Machine Learning is now available as an e-book or printed copy from Bentham Books and Amazon.